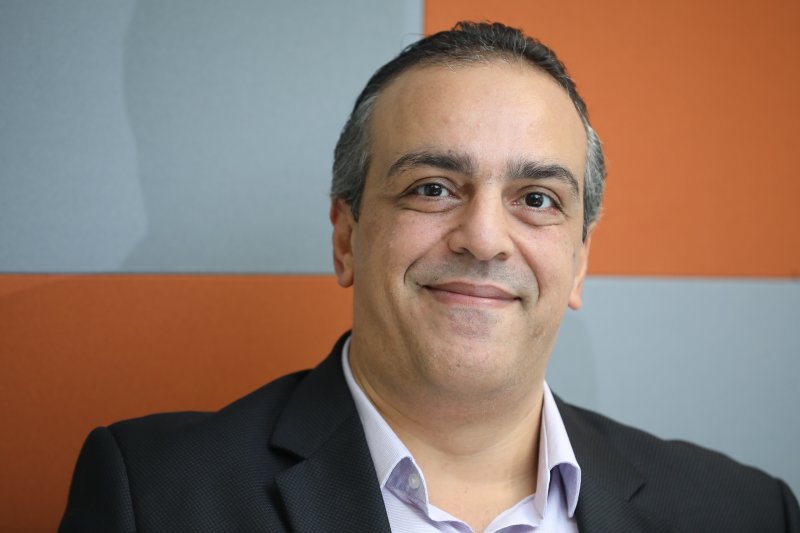
“Most AI models are more like black boxes. Data goes into the black box, and even data scientists struggle to explain how they reach conclusions.” IBM’s artificial intelligence leader Tarek Saeed believes Big Blue now has the answer to some of AI’s most confusing aspects. “We’ve provided an ability to explain why a model comes up with results,” he says. “This is critical for different industries.”
IBM has introduced technology that provides increased transparency into AI – a software service that automatically detects bias and explains how AI makes decisions as they are being made, which runs on the IBM Cloud. The “fully automated” software service explains decision-making and detects bias in AI models at runtime, capturing potentially unfair outcomes as they occur. Importantly, it also automatically recommends data to add to the model to help mitigate any bias it has detected.
Saeed works with the worldwide team at IBM to help progress ideas from global research to the Middle East, as well as working with the firm’s local practice that helps clients to build roadmaps and POCs around AI. “IBM started focusing on AI research in 2006,” he says. “We looked at its first application through Watson in healthcare, and we commercialised it in 2014. Even then. we faced challenges, and at that stage, people were not really talking about AI. Applying this new technology wasn’t something that had been done before.”
However, these early challenges proved to be an important learning experience. “The main benefit that we got from that is that we learned a lot quickly, and it gave us the opportunity to improve our AI capabilities, and that has given us a big advantage,” Saeed says. “It’s not just about technology – implementing and maintaining AI in a sustainable fashion is as big a challenge.”
IBM’s new Trust and Transparency capabilities work with models built from a variety of machine learning frameworks and AI-build environments such as Watson, Tensorflow, SparkML, AWS SageMaker, and AzureML, enabling the monitoring of decision factors of “any business workflow”.
“The biggest challenge with AI and machine learning is detecting things like bias and ensuring that outcomes and results from different models are not biased,” Saeed says. “Data inherently has bias. For example, when you try to apply for bank loan, data focuses on factors like age groups. A customer may not be part of a desirable loan group. It could also focus on minorities. The trick is to have technology, tooling or capabilities to address these flaws.”
Explanations within Trust and Transparency show which factors have weighted AI-driven decisions in one direction as opposed to another, as well as the confidence in the recommendation, and the factors behind that confidence. The records of the model’s accuracy, performance and fairness, and the lineage of the AI systems, are traced and recalled for customer service, regulatory or compliance reasons.
The developments come on the back of new research by IBM’s Institute for Business Value, which has revealed that while 82% of enterprises and 93% of “high-performing enterprises” are considering AI deployments, 60% fear liability issues and 63% lack the in-house talent to confidently manage the technology.
According to the Value AI 2018 Report, there is a significant shift underway in how business leaders look at AI’s potential to drive business value and revenue growth. CEO’s interviewed in the study believe the greatest value in AI adoption to be in IT, information security, innovation, customer service, and risk management.
Saeed believes there are already several clear use cases for the Trust and Transparency technology, and healthcare is one such industry where he believes transparency around AI-driven decisions will be essential. “If AI is used to help give a doctor advice, the doctor needs to explain how the diagnosis has been reached,” he says. “You need to be able to check the viability of models and predictions as they are being deployed.”
He also cites the way that IBM has used AI in attempting to predict outcomes of crime, but again, this has encountered issues with trust. “We’ve done this in some US precincts, but the data bias focuses more on areas with minorities,” he says. “Bias is embedded in society and we want to remove that. We need the tools, technology and capabilities to ensure we actively address that. Otherwise, bias will remain in the data.”
According to Saeed, given AI’s relative immaturity in the enterprise, it is important to ensure that the appropriate support services are on offer to ensure its successful delivery. That thinking is also a contributing factor in the firm’s decision to create tools for the open source community that can help to accelerate the pace of AI development. “Most organisations are looking to move from the AI experimentation to deployment phase,” Saeed says. “IBM foresees a lot of challenges when they reach this stage.”
IBM’s research division will release an AI bias detection and mitigation toolkit into the open source community. The AI Fairness 360 toolkit – a library of algorithms, code and tutorials aims to give academics, researchers, and data scientists the tools and knowledge to integrate bias detection as they build and deploy machine learning models. While other open-source resources have focused solely on checking for bias in training data, the AI Fairness 360 toolkit will help check for and mitigate bias in AI models.
Beyond the issues of trust and transparency, Saeed believes there are two major issues that must be resolved with AI. “Data and its readiness for AI is the main issue,” he says. “AI is like a car – data is its gas. Data is a challenge, both in its quality and completeness. Organisations now have the opportunity to initiate a data transformation. The other challenge is talent. It’s about upskilling young people to understand and use the technology.”