Ammar Enaya, regional director – Middle East, Turkey & North Africa (METNA), Vectra, discusses the benefits of making data science the foundation of new cybersecurity models.
Cyber-attacks are no longer simple smash-and-grab jobs driven by pre-programmed malware. They are controlled by highly skilled, creative and intelligent humans. Ongoing coordination allows a human attacker to progressively learn more about the target network, adapt to any defensive measures, and advance the attack over time.
But while attacks have made an evolutionary leap in complexity, security defences have not. Signatures are the bedrock of traditional security technology and are written to identify exploits, malicious URLs and known malware as they seek to penetrate and gain an initial foothold inside the organisation. Signatures can quickly identify, and block known threats at scale. However, their weakness is that they are inherently reductive — they reduce a known threat to its simplest fingerprint in order to give a single yes or no answer within microseconds to avoid slowing the flow of application traffic. This reductive focus on immediate and simple answers has created an advantage for attackers who are willing to adapt.
However, it is the persistence of the ongoing attack that has truly turned the tables. Once an organisation’s outer defences are compromised, attackers can blend in with the network, progressively spy, and spread deeper, until they find high-value assets to steal or destroy. This process typically involves multiple compromised hosts, a variety of common tools and protocols that blend into the noise of everyday communications, and the theft and misuse of valid user credentials.
The important point is that the threat itself is ongoing while attackers evolve their operations and adapt over time. The reductive nature of signatures that identify threats at the atomic level is particularly ill-equipped for recognising the more complex chemistry going on around them. This intelligence gap is precisely why a new security model for threat detection is so vital.
Coarse-grained detections with a long shelf life
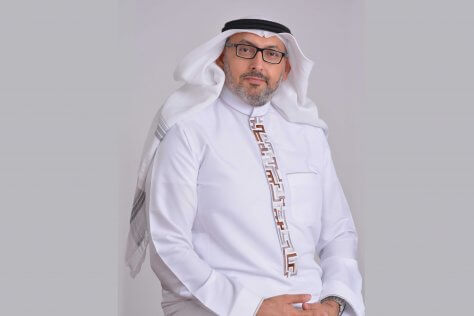
Detections that use traditional signatures become obsolete when attackers adapt by moving to a new domain or by adding a few bits to known malware, so signatures no longer match it. This gives them a first-mover advantage where even the most trivial changes keep attackers several steps ahead of defenders.
One of the core goals of the new threat detection model is to deliver detections that remain valid for long periods of time. This requires a shift from fingerprinting every individual instance of a threat to recognising the fundamental attack characteristics that every threat has in common. When applied to packet-level traffic, data science and machine learning become extremely powerful tools to identify the fundamental characteristics that distinguish threats from normal traffic.
Focus on attacker actions and behaviours
Traditional detection models attempt to find snippets of exploit code, a known sample of malware or a malicious domain. This leads to an intractable job of constantly finding and fingerprinting an infinite number of malicious occurrences. The task is never-ending, and attackers always remain steps ahead by using a new exploit.
To break this cycle, the new threat detection model shifts the focus from trying to name all possible bad ‘things’ to identifying the unique indicators of attack behaviours and actions. In other words, the goal shifts from identifying what a ‘thing’ is, to identifying what the ‘thing’ does. Although attackers can hide their threats by making slight changes to malware or buying a new domain, the actions and objectives of an attack are always the same.
By focusing on attack behaviours, defenders can fight and win the asymmetric cybersecurity war by shifting the math of security back in their favour. Instead of using thousands of signatures to find every variant of a threat, they can focus on a few dozen key behaviours that attackers must perform in order to succeed.
Recognise threats over time
One of the most recognisable traits of modern network data breaches is that they evolve over time. This low-and-slow approach has become standard operating procedure for sophisticated attacks, and for good reason. Traditional security suffers from short-term memory and a post-breach form of perfect amnesia.
By understanding prevalent attacker Tactics, Techniques and Procedures (TTPs) from evidence-based sources like the Mitre ATT&CK framework, we can understand the steps that future possible attacks will use, and develop novel techniques to identify them.
The new threat detection model recognises threats in real-time and identifies the signs of attacks that evolve over time. One does not preclude the other. For example, small temporal anomalies and cadences within a network session can help reveal hidden tunnels and remote access tools used by attackers. Conversely, recognising when an employee’s credentials have been compromised may require learning the user’s normal behaviours over a period of days, weeks and months. While the time scale can vary, both cases require a keen understanding of threats in relation to time.
Recognise attacks, not just techniques
In order to provide value, security must identify real business risks to an organisation and not simply deliver a list of alerts. This requires security solutions to understand how individual events are interconnected and the impact those threats have on an organisation’s assets. This necessitates a combination of threat context and organisational context. The ability to connect the dots between phases of an attack is precisely what distinguishes a targeted attack from the stream of commodity threats that inundate networks on a daily basis.
Why Data Science?
Data science represents a fundamental shift in security. Unlike a signature-based approach that delivers a 1-for-1 mapping of threats to countermeasures, data science uses the collective learning of all threats observed in the past to proactively identify new ones that haven’t been seen before.
Long term, it is essential to understand what, when, why and how. Actual knowledge and intelligence is far more advantageous when evaluating and solving new problems that have not been encountered before. This is a critically important distinction when using data science to detect threats. For the traditional model to work, all of the answers must be known ahead of time. Data science expects to be asked real questions and applies collective learning to evaluate an unknown.
As such, the newest, most advanced threat detection model combines a wide array of industry-leading intelligence and detection techniques to see threats from all angles in real time. It represents a new, more effective and highly proficient detection methodology that leverages data science to detect threats that are missed by traditional security models.